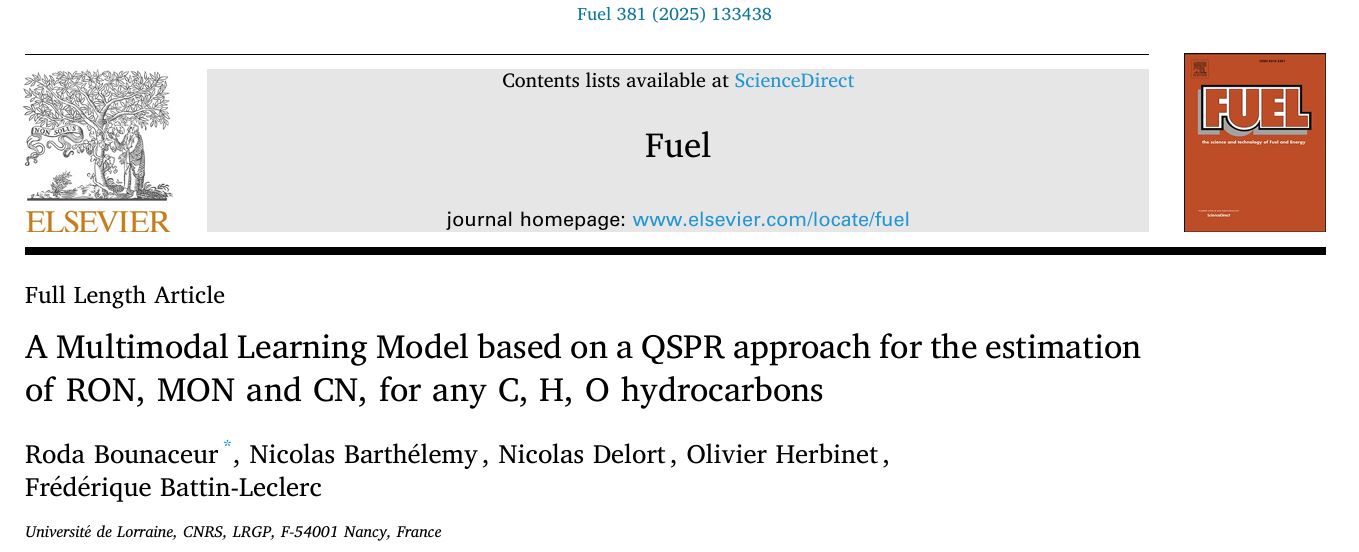
With the increasing demand in alternative fuels and the use of biomass as the feedstock for the production of fuel commodities, a wider range of oxygenated hydrocarbons as fuel additives needs to be considered. Consequently, the development of robust method for the prediction of criterium as Research Octane Number (RON), Motor Octane Number (MON) or Cetane Number (CN), will play a crucial role in the characterization of novel fuels. In this paper we proposed a robust deep-learning model based on a Quantitative Structure−Property Relationship (QSPR) approach for the estimation of RON, MON and CN of any C, H, O, N molecules.
We develop a multimodal learning model from a combination of two types of data and based on an Artificial Neural Network. The Mordred algorithm has been used in order the determine 457 descriptors to characterize any hydrocarbons. These numerical values represent the first type of data considered in the present study.
To consider the effect of mesomerism or chirality of molecules the InChiKey notation has been used. This notation, composed by 27 letters, represent the second type of data and are considered as text data. To encode textual variables into numeric data, we have used a Word Embedding method.
The final model has been tested with success on a large set of experimental data and compared with five recent learning models: GNN, ANN, GPR, DLMO and PIGNN. The methodology of GNN (Graph Neural Networks) model is based on the architecture of the molecule, that of the ANN (Artificial Neural Networks) is based on a limited number of chemical groups, whereas the GPR (Gaussian Process Regression) model is mainly based on Joback groups. The two recent methodologies DLMO (Deep Learning Mixing Operator) and PIGNN (Physics-Informed Graph Neural Network) are based on more sophisticated algorithms. The full comparison and the several tests show a very robust and predictive ability of our newly proposed multimodal learning model.
Hit the button in order to get an access to the web frontend of the multimodal neural network-based tool for computation of RON, MON and CN of any H, C, O, N hydrocarbons.